Talk Info
Updating...
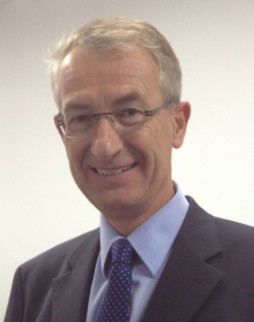
Milan Sonka (Iowa Institute for Biomedical Imaging,University of Iowa)
Title: Prediction of Cardiac Allograft Failure: Quantitative Analysis of Coronary OCT Wall Morphology in Heart-Transplant Patients
Abstract: Cardiac Allograft Vasculopathy (CAV) is a frequent complication of heart transplantation
(HTx). To help identify patients destined for cardiac graft failure, early determination of
layer-specific coronary wall thickening is of major importance. For patients destined for CAV
complications of HTx, therapy must be initiated early to be effective. Therefore, early diagnosis of
CAV is paramount to minimize CAV-related graft failures. Once CAV causes allograft dysfunction,
the only long-term therapeutic solution is a re-transplantation.
Our work reports methods for and validation results of quantitative analysis of coronary OCT images
in HTx patients that will facilitate creating predictive models identifying which individual HTx
patient is pre-destined for cardiac allograft vasculopathy and thus a candidate for aggressive therapy
early after the heart transplant surgery. Our new quantitative analysis of coronary wall morphology
offers performance statistically indistinguishable (p=NS) from that of expert tracing. The reported
approach is fast, efficient, and facilitates accurate layer-specific quantification of coronary wall
thickness changes after HTx and serves as the validated pre-requisite to precision medicine
approaches to CAV failure prediction.
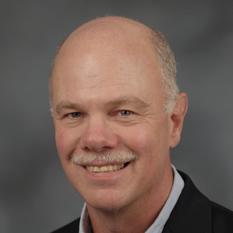
James S.Duncan (School of Engineering & Applied Science, Yale University)
Title: Left Ventricular Deformation Analysis from 4D Echocardiography
Abstract: Myocardial infarction (MI) remains a leading cause of morbidity and death in many countries. Acute MI causes regional dysfunction, which places remote areas of the heart at a mechanical disadvantage resulting in long term adverse left ventricular (LV) remodeling and complicating congestive heart failure (CHF). Echocardiography is a clinically established, cost-effective technique for detecting and characterizing coronary artery disease and myocardial injury by imaging the left ventricle (LV) of the heart. Our laboratory has been working on the development of an image analysis system to derive quantitative 4D (three spatial dimensions + time) echocardiographic (4DE) deformation measures (i.e. LV strain) for use in diagnosis and therapy planning. These measures can localize and quantify the extent and severity of LV myocardial injury and reveal ischemic regions.
In this talk, the image analysis systemthat combines displacement information from shape tracking of myocardial boundaries (derived from B-mode echocardiographic data) with mid-wall displacements from radio-frequency-based ultrasound speckle tracking to estimate myocardial strain will be discussed. Our current implementation is based on Bayesian analysis and radial basis functions for integrating information. A new robust approach for estimating improved dense displacement measures based on an innovative data-driven, deep feed-forward, neural network architecture that employs domain adaptation between data from labeled, carefully-constructed synthetic models of physiology and echocardiographic image formation (i.e. with ground truth), and data from unlabeled noisy in vivo porcine or human echocardiography (missing or very limited ground truth) will also be described. Included in this will be an overview of our current strategy for LV surface segmentation via patch-based dictionary learning, our latest graph-based flow network ideas for surface shape tracking and early work on the use of Siamese neural networks for intensity-based patch matching. Test results on LV strain will be presented from synthetic and in vivo4DE image sequence data, including a comparison to strains derived from MR tagging.
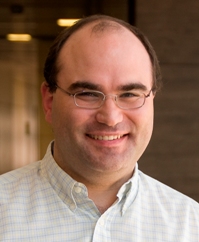
Alejandro Frangi (Electronic & Electrical Engineering Department,University of Sheffield)
Title: Precision Imaging in Cerebrovascular Diagnosis and Interventional Planning
Abstract: Current technological progress in multidimensional and multimodal acquisition of biomedical data enables detailed investigation of the individual health status that should underpin improved patient diagnosis and treatment outcome. However, the abundance of biomedical information has not always been translated directly in improved healthcare. It rather increases the current information deluge and desperately calls for more holistic ways to analyse and assimilate patient data in an effective manner. The Virtual Physiological Human aims at developing the framework and tools that would ultimately enable such integrated investigation of the human body and rendering methods for personalized and predictive medicine. This lecture will focus on and illustrate two specific aspects: a) how the integration of biomedical imaging and sensing, signal and image computing and computational physiology are essential components in addressing this personalized, predictive and integrative healthcare challenge, and b) how such principles could be put at work to address specific clinical questions in the cardiovascular domain. Finally, this talk will also underline the important role of model validation as a key to translational success and how such validations span from technical validation of specific modelling components to clinical assessment of the effectiveness of the proposed tools.
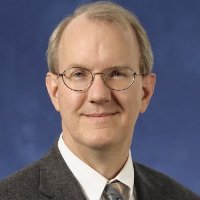
Jerry Prince (Johns Hopkins University, US)
Title: Image Synthesis and its Applications in Medical Image Analysis
Abtract: Image synthesis methods can take acquired images and produce images with a contrast or
modality that was not imaged. While not yet trusted for clinical use, these methods are starting to
prove invaluable in medical image analysis. For example, in PET-MR scanners, CT images are
synthesized from the acquired MR images and used for attenuation correction in PET reconstruction.
Synthesized images can be used to replace missing contrasts in standard algorithms pipelines images
were not acquired or were corrupted by noise or artifacts. Image synthesis can be used to normalize
image intensities in MR images, which lack a standard intensity scale. This can be very useful in
multi-center trials involving scanners from different manufacturers, hardware, and pulse sequence
implementations. Image synthesis can be used to improve multi-modal registration by synthesizing
images so that same-modality similarity metrics can be used instead of mutual information. Image
synthesis can also be used to improve resolution--i.e., to provide super-resolution. Finally, image
synthesis can be used to normalize longitudinal image acquisitions that were acquired over time and
have data acquired with different resolutions, pulse sequences, and even scanner strengths. Different
image synthesis approache will be described, starting from a historical perspective and ending with
the most modern approaches that are under development today. Different applications will be
described and demonstrated to illustrate the great utility and potential of these methods.
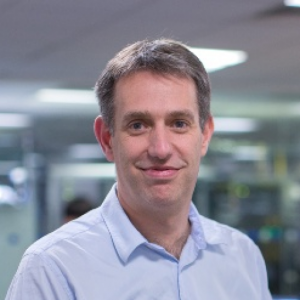
Daniel Rueckert (Department of Computing, Imperial College London)
Title: Learning clinically useful information from medical images
Abtract:This talk will focus on the use of deep learning techniques for the discovery and
quantification of clinically useful information from medical images. The talk will describe how deep
learning can be used for the reconstruction of medical images from undersampled data,
image super-resolution, image segmentation and image classification. We will also show the
clinical utility of applications of deep learning for the interpretation of medical images in
applications such as brain tumour segmentation, cardiac image analysis and applications in
abdominal imaging.
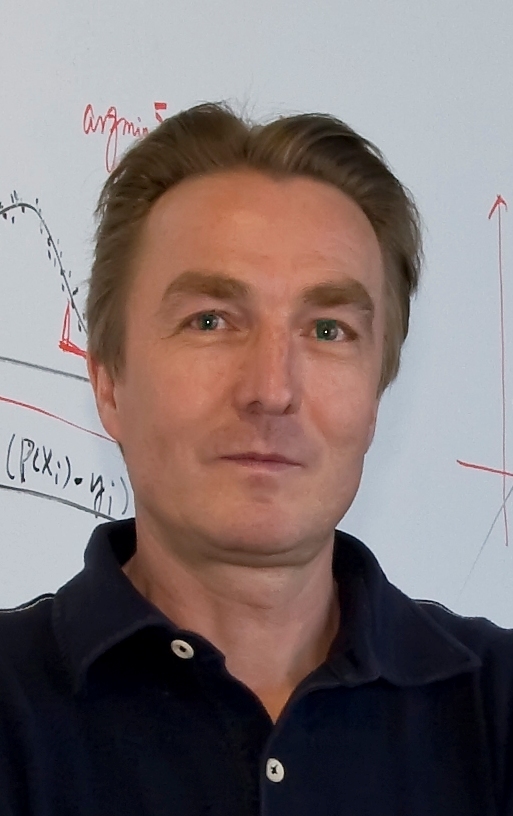
Guido Gerig (NYU Tandon School of Engineering)
Title: Studying Growth and Disease Trajectories from Longitudinal Imaging: Challenges and Oportunities
Abstract: Clinical assessment routinely uses terms such as development, growth trajectory, aging,
degeneration, disease progress, recovery or prediction. This terminology inherently carries the
aspect of dynamic processes, suggesting that measurements of dynamic spatiotemporal changes may
provide information not available from single snapshots in time.
Image processing of temporal series of 3-D data embedding time-varying anatomical objects and
functional measures requires a new class of analysis methods and tools that makes use of the inherent
correlation and causality of repeated acquisitions. This talk will discuss progress in the development
of advanced 4-D image and shape analysis methodologies that carry the notion of linear and
nonlinear regression, now applied to complex, high-dimensional data such as images,
image-derived shapes and structures, or a combination thereof. Methods include joint segmentation
of serial 3D data enforcing. temporal consistency, building of 4-D models of tissue diffusivity via
longitudinal diffusion imaging, and 4-D shape models. We will demonstrate that statistical concepts
of longitudinal data analysis such as linear and nonlinear mixed-effect modeling, commonly applied
to univariate or low-dimensional data, can be extended to structures and shapes modeled from
longitudinal image data. We will show results from ongoing clinical studies such as analysis of early
brain growth in subjects at risk for autism, analysis of neurodegeneration in normal aging and
Huntington's disease, and quantitative assessment of recovery in severe TBI.
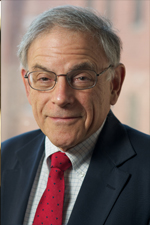
Murray H.Loew (George Washington University, US)
Title: Biomedical applications of hyperspectral imaging: the example of cardiac ablation
Abstract: Imaging across the electromagnetic spectrum, both passive and active, has provided insights in many areas, from large scale (remote sensing of the earth) to small (visualizing cell activity). With emphasis on biomedical applications, this talk presents some of the basic methods for acquisition and representation of images that span various parts of the spectrum, and for classifying regions in the images. A promising application is the treatment of atrial fibrillation by microwave ablation of the sources of dyssynchronous electrical activity. By taking advantage of the spectral properties of NADH and of the underlying tissue, we can determine whether ablation has been successful. This raises the prospect of enabling the clinician to assess in real-time the status of the ablation and thus to reduce the number of patients requiring subsequent re-treatment. We discuss the development of a hyperspectral imaging catheter and the accompanying analysis methods that are expected to yield a tool for intraoperative treatment and monitoring.
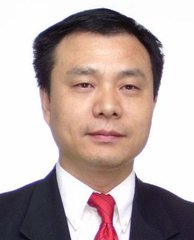
Jie Tian (Institution of Automation of CAS, China)
Title: Multimodality Molecular Imaging from Preclinical Research to Clinical Translation
Abstract: Cutting-edge technologies of optical molecular imaging have ushered new frontiers in cancer research, clinical translation and medical practice, as evidenced by recent advances in optical multimodality imaging, Cerenkov luminescence imaging, and optical image-guided surgeries. New
abilities allow in vivo cancer imaging at sensitivity and accuracy unprecedented for conventional
imaging approaches. The visualization of cellular and molecular behaviors and events within tumors
in living subjects are improving the deeper understanding of tumors at a systems level. These
advances are rapidly used to acquire tumor-to-tumor molecular heterogeneity, dynamically
and quantitatively, as well as to achieve more effective therapeutic interventions with real-time
imaging assistance. In the era of molecular imaging, optical technologies hold great promise to
facilitate the development of highly sensitive cancer diagnosis and personalized patient treatment,
which is one of the ultimate goals of precision medicine.
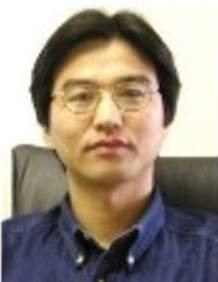
Dinggang Shen (The University of North Carlina at Chapel Hill)
Title: Deep Learning for Medical Image Analysis
Abstract: This talk will discuss some of our recently developed deep learning methods for various
neuroimaging applications. Specifically, 1) in neuroimaging analysis, we have developed an
automatic brain measurement method for the first-year brain images with the goal of early detection
of autism such as before 1-year-old. This effort is aligned with our recently awarded Baby
Connectom Project (BCP) (where I serve as Co-PI), which will acquire MR images and behavioral
assessments from typically developing children, from birth to five years of age. Besides, we have
also developed a novel landmark-based deep learning method for early diagnosis of Alzheimer’s
Disease (AD) with the goal of potential early treatment. 2) In image synthesis, we have developed
a cascaded 3D CNN for reconstructing 7T-like MRI from 3T MRI for simultaneously enhancing
image quality and tissue segmentation. Also, we have developed a novel Generative Adversarial
Networks (GAN) based technique to estimate CT from MRI, for helping MRI-based cancer
radiotherapy. All these techniques will be introduced in this talk, for the goal of early diagnosis of
brain disorders.
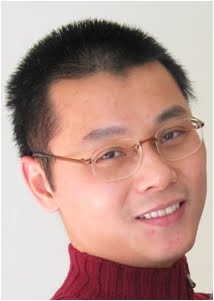
S. Kevin Zhou (Siemens Healthineers, Siemens Corporate Research)
Title: Deep Learning & Beyond: Medical Image Recognition, Segmentation and Parsing
Abstract: The "Machine learning + Knolwedge" approaches, which combine meachine learning with domain knowledge, enable us to achieve start-of-the-art performances for many tasks of medical image recognition, segmentation and parsing. In this talk, we first present real success stories of such approaches. Then, we proceed to elaborate deep learning, a special, mighty type of machine learning method, and review its recent advances. We conclude with several latest "DL & Beyond" works.
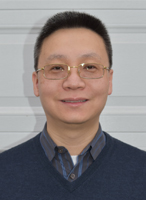
Le Lu (National institute of health, the USA)
Title: Building Truly Large-scale Medical Image Databases: Deep Label Discovery And Open-ended Recognition
Abstract: The recent rapid and tremendous success of deep neural networks on many challenging
computer vision tasks derives from the accessibility of the well-annotated ImageNet and PASCAL
VOC datasets. Nevertheless, unsupervised image categorization (that is, without ground-truth
labeling) is much less investigated, critically important, and difficult when annotations are extremely
hard to obtain in the conventional way of "Google Search" + crowd sourcing (exactly how ImageNet
was constructed). We'll present recent work on building two truly large-scale radiology image
databases at NIH to boost the development in this important domain. The first one is a chest X-ray
database of 110,000+ images from 30,000+ patients, where the image labels were obtained by
sophisticated natural language processing-based text mining and the image recognition benchmarks
were conducted using weakly supervised deep learning. The other database contains about 216,000
CT/MRI images with key medical findings from 61,845 unique patients, where a new looped deep
pseudo-task optimization framework is proposed for joint mining of deep CNN features and image
labels. Both medical image databases will be released to the public.
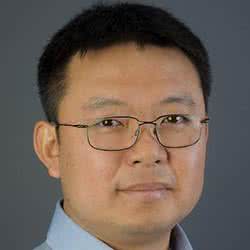
Hao F Zhang (Department of Biomedical Engineering, Northwestern University, US)
Title: Visible-light optical coherence tomography: seeing retinal functions and beyond
Abstract: As the elderly populations of Europe, China and the U.S. grow, the prevalence of major
ophthalmic disorders such as glaucoma, age-related macular degeneration (AMD), and diabetic
retinopathy are also expected to increase, fueling new demand for improved diagnostic and surgical
systems to help physicians manage and treat these diseases. Optical coherence tomography (OCT)
devices currently represent the gold standard for ophthalmic diagnostics, providing high-resolution
imaging and proven clinical benefit in improving the patient’s quality of life. Despite the success of
OCT, however, the full potential of this imaging modality has yet to be realized. While other imaging
methods such as MRI and PET have been revolutionized with the development of functional imaging
(e.g. fMRI), functional OCT remains an emerging technology. Visible-light OCT (Vis-OCT)
represents a cutting-edge functional OCT imaging technique that aims to dramatically improve the
diagnostic capabilities and clinical benefit of OCT in ophthalmology. Vis-OCT is currently the only
OCT technology capable of combining both high-resolution structural imaging with precise
measurements of metabolic activity, such as retinal oxygen saturation and retinal blood flow. Using
dual band scanning with visible light and NIR light wavelengths, Vis-OCT represents a next
generation functional OCT tool with the potential to fundamentally change how ophthalmologist use
OCT in the diagnosis, treatment and monitoring of numerous major ocular disorders.
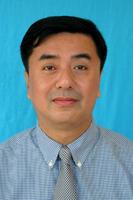
Xun Xu (Shanghai General Hospita, China)
Title: How does OCTA change the clinical practice
Optical coherence tomography angiography (OCTA) is a non-invasive, safer, and faster imaging technique, which can acquire more clear retinal vascular images without contrast agent injection and easy to be repeated.
The talk gives clinical examples on how OCTA has changed the clinical practice in four aspects: (1) Changing the understanding of the disease. (2) Helping to diagnose disease and differentiate diagnosis. (3) Optimizing the process of diagnosing the disease. (4) Redesigning the treatment and the follow-up plan.
The talk also includes review of recent studies on retinal vascular diseases based on OCTA, such as early diagnosis of retinal vascular disease based on three-dimensional convolution network model, vascular density analysis based on OCTA image, OCTA-based microvascular tumor detection, automatic detection and quantitative analysis of abnormal blood vessel based on OCTA.
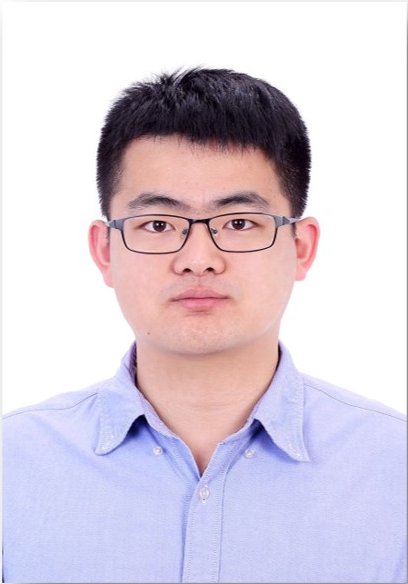
Hao Chen (The Chinese University of Hong Kong,Imsight Medical Technology Inc.)
Title: 3D Deep Learning and Its Application to Volumetric Medical Image Analysis
Abstract: Deep learning represents data with multiple levels of abstraction and has dramatically improved the state-of-the-art in many domains including speech recognition, visual object recognition and natural language processing. Despite its breakthroughs in above domains, itsapplication to volumetric medical image analysis remains to be fully explored. This talk will share our recent studies on developing state-of-the-art 3D deep learning methods including fully convolutional networks, deeply supervised nets, voxelwise and dense residual networks for medical image analysis, with an in-depth dive into several medical applications.
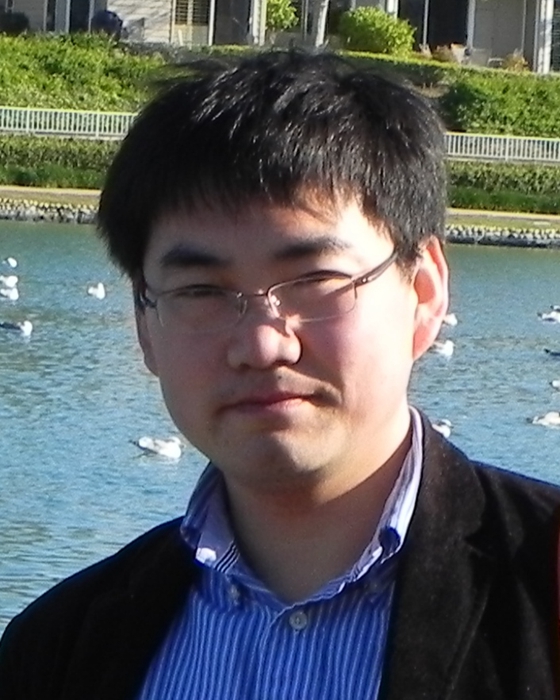
Yue Gao (School of software, Tsinghua University, China)
Title: Hypergraph Structure Learning and Its Applications in Medical Image Analysis
Abstract: Hypergraph is a general graph structure and has been widely applied in data classification,
image segmentation and retrieval due to its superior performance on high-order correlation
modelling. In recent years, extensive research efforts have been dedicated to hypergraph based
learning methods. In this presentation, we will first introduce the hypergraph construction methods,
considering both single modality and multi-modality scenarios. After that, we will present the
learning methods on hypergraph structure, from traditional transductive learning to hypergraph
structure learning, including the information about vertex, hyperedge and multi-hypergraphs. Finally,
we will introduce the applications of hypergraph structure learning in medical image analysis.
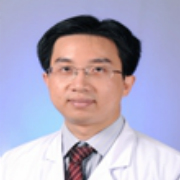
Jin Yuan (Zhongshan Ophthalmic Center, Sun Yat-Sen University)
Title: Conjunctival Microvasculature & Microcirculation as new indicators in Dry Eye Inflammation Evaluation
Abstract: This study was conducted to determine blood flow velocities and non-invasive bulbar conjunctival microvascular perfusion maps (nMPMs) to characterize the morphometric and hemodynamic features of bulbar conjunctiva on dry eye disease.
Normal subjects and dry eye (DE) patients were prospectively recruited with the conjunctival microvasculation using a novel Functional Slit-lamp Biomicroscope (FSLB). The temporal bulbar conjunctiva were imaged and analyzed. Main variables including blood flow velocities, nMPMs and vessel diameter in the two groups were compared with the software developed by our study group.
The bulbar blood flow velocity was 0.59 ± 0.09 mm/s in DE group and 0.47 ± 0.12 in normal group (P < 0.001). And the vessels density (Dbox) was higher in DE patients (1.65 ± 0.04) than normal controls (1.60 ± 0.07, P < 0.05). Moreover, the vessel diameter was larger in DE group (21.78 ± 1.78 μm) compared with normal controls (17.92 ± 2.24 μm, P <0.001). Besides, Dbox positively associated with ocular surface disease index (OSDI) (r = 0.442, P = 0.027).
Microvascular abnormalities were found in the bulbar conjunctiva of DE patients, including increased blood flow velocity, vessel density and larger vessel diameter.
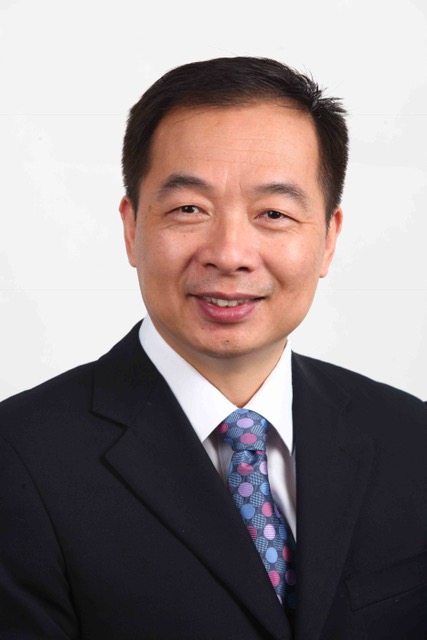
Qinghuai Liu (Department of Ophthalmology, Jiangsu Province Hospital, China)
Title: A method for auto-segmentation and analysis of hyper-reflective foci and hard exudate in patients with diabetic retinopathy
Purpose: To investigate the correlations between hyper-reflective foci and hard exudates in patients with nonproliferative diabetic retinopathy (NPDR) and proliferative diabetic retinopathy (PDR) by spectral domain optical coherence tomography (SDOCT) images.
Methods: Hyper-reflective foci in retinal SD OCT images were automatically detected by the developed algorithm. Then, the cropped CFP images generated by the semi-automatic registration method were automatically segmented for the hard exudates and corrected by the experienced clinical ophthalmologist. Finally, a set of 5 quantitative imaging features were automatically extracted from SD OCT images, which were used for investigating the correlations of hyper-reflective foci and hard exudates and predicting the severity of diabetic retinopathy.
Results: Experimental results demonstrated the positive correlations in area and amount between hard exudates and hyper-reflective foci at different stages of diabetic retinopathy, with statistical significance (all p < 0.05). In addition, the area and amount can be taken as potential discriminant indicators of the severity of diabetic retinopathy
Conclusion:Our work appears to be a novel approach to discuss the correlations between hyper-reflective foci and hard exudates; and a model is built to objectively predict the severity of DR based on quantitative imaging features.
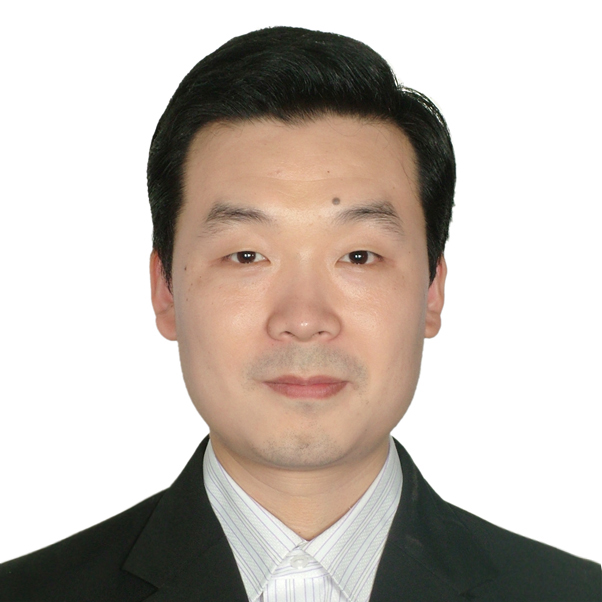
Yu Qiao (Shenzhen Advanced Technology Research Institute, Chinese Academy of Science, China)
Title: Deep Vision for Human Vision
Recent studies show that deep neural networks achieve tremendous successes in various computer vision problems. The deep computer vision techniques are partly inspired by human vision mechanism. On the other hand, deep vision techniques provide novel and effective tools for the understanding, disease diagnosis, and enhancement of human vision system. This talks will report our recent progresses on developing novel deep models for analyzing vision field, OCT and fundus with application in eye diseases diagnosis. We will also report our efforts in transferring these techniques for clinic practice.